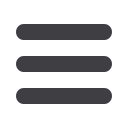
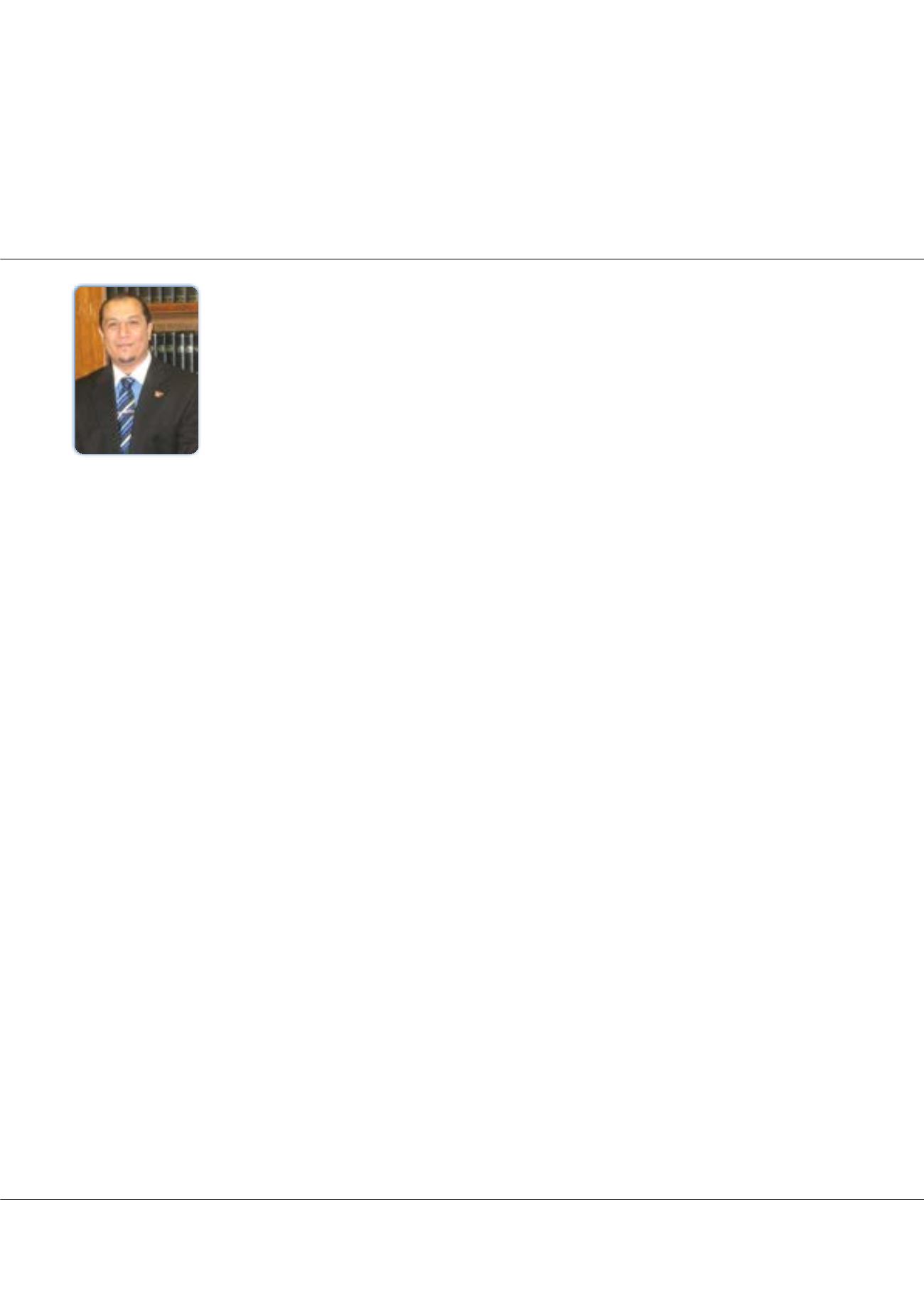
Page 23
Notes:
conference
series
.com
Volume 8, Issue 5 (Suppl)
J Biom Biostat, an open access journal
ISSN: 2155-6180
Biostatisitcs 2017
November 13-14, 2017
November 13-14, 2017 | Atlanta, USA
6
th
International Conference on
Biostatistics and Bioinformatics
Model robust profile monitoring for the generalized linear mixed model for phase I analysis
T
here are so many applications for detecting the changes in the relationship between the response variable and explanatory
variable (s) may be the most important consideration rather than detecting the changes in univariate or multivariate
quality characteristics. This relationship between the response variable and one or more explanatory variables is called a profile.
The act of using various techniques to statistically monitor the process or product profiles is known as profile monitoring. The
study introduces two mixed model methods to monitor profiles from the exponential family: a nonparametric (NP) regression
method based on the penalized spline regression technique and a semi-parametric (SP) method (Model robust profile
monitoring for the generalized linear mixed model (MRGLMM)) which combines the advantages of both the parametric and
nonparametric methods. A correctly specified parametric (P) model will have the most power in detecting the profile shift,
while a NP method can give improved performance for any type of profile. The MRGLMM method gives results similar to the
P method when P model is correctly specified and it gives results similar to the NP method if the proposed P model is badly
misspecified. The MRGLMM method gives results that are superior to either the P method or the NP method if the P model
provides some useful information regarding profile behavior. Thus, the MRGLMMmethod is robust to model misspecification.
The performances of P, NP and MRGLMM methods are compared through a simulation study using binary data.
Biography
Abdel-Salam Gomaa holds BS and MS (2004) degrees in Statistics from Cairo University and MS (2006) and PhD (2009) degrees in Statistics from Virginia Poly-
technic Institute and State University (Virginia Tech, USA). Prior to joining Qatar University as an Assistant Professor and a Coordinator of the Statistical Consulting
Unit and Coordinator for the Statistics Programs, he taught at Faculty of Economics and Political Science (Cairo University), Virginia Tech, and Oklahoma State
University. Also, he worked at JPMorgan Chase Co. as Assistant Vice President in Mortgage Banking and Business Banking Risk Management Sectors. He has
published several research papers and delivered numerous talks and workshops. He has awarded couples of the highest prestige awards such as Teaching Ex-
cellence from Virginia Tech, Academic Excellence Award, Freud International Award, and Mary G Natrella Scholarship from American Statistical Association (ASA)
and American Society for Quality (ASQ), for outstanding graduate study of the theory and application of Quality Control, Quality Assurance, Quality Improvement,
and Total Quality Management. He is a Member of the ASQ and ASA.
abdo@qu.edu.qaAbdel-Salam Gomaa
Qatar University, Qatar
Abdel-Salam Gomaa, J Biom Biostat 2017, 8:5 (Suppl)
DOI: 10.4172/2155-6180-C1-004